about
I graduated Summa Cum Laude from Arizona State in 2021 with a BS in mathematics, mentored in data-driven dynamical systems under Yang Kuang, Eric Kostelich and Chris K.R.T. Jones (see my 2020 paper on applying data assimilation techniques to forecast metastasized prostate cancer growth). I have an interest in fusing dynamical models with real data using data assimilation for improved predictive modeling (see the 2021 paper with NORCE scientist Geir Evensen and JCSDA/UCAR project scientist Christian Sampson on ensemble data assimilation of a SEIR-based covid model.)
I graduated 2023 from Johns Hopkins with a MSE in applied mathematics, mentored by Distinguished Professor Yannis Kevrekidis in data-driven dynamical systems and model reduction (see master’s thesis on center manifold learning). Some other notable collaborators of mine are Dr. Tin Phan of Los Alamos’ Theoretical Biology and Biophysics lab, and Dr. Emmanuel Fleurantin of George Mason University.
I am incredibly interested in the theory of dynamical systems and high-dimensional geometry. I am also interested in applications to data-driven dynamics, and data science - particularly model reduction, and sparse recovery. Also, I am incredibly competitive - I competed in the USPA as an amateur powerlifter in the 242 lbs class for many years where I was mentored by Arizona elite master lifter Tim Sparkes. I also enjoy watching the Texas Rangers, so I sometimes incorporate competition/sports into data science projects (see my R work on analyzing MLB player salary trends using a random forest architecture.)
work
Please reach out if there are any questions, critiques, or if interested in collaborations.
Personal
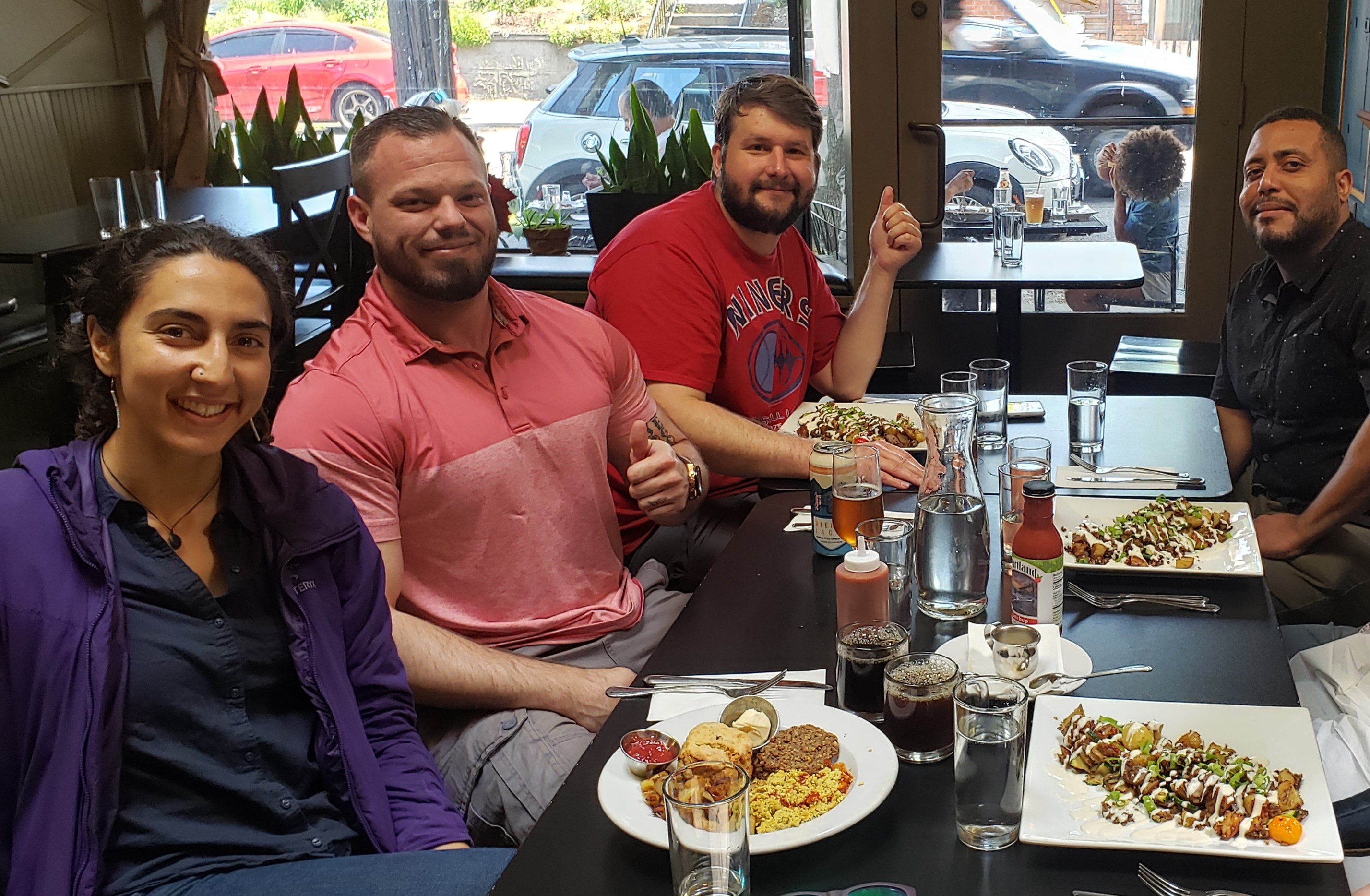
at SIAM's DS23. Left to right, Olivia Chandresekhar (Los Alamos), Justin Bennett, Christian Sampson (UCAR, JCSDA), and Emmanuel Fleurantin (GMU).
Projects/Other work
- Data Science
- On The Fast Fourier Transform
- Sparse recovery using BP and OMP
- Baseball salary predictor selection using R
- Differential expression and supervised learning
- Model selection presentation for used car prices
- Dynamical Systems
- Stoichiometric dynamics of ant-fungus mutualism
- Master Thesis
- Center manifold learning
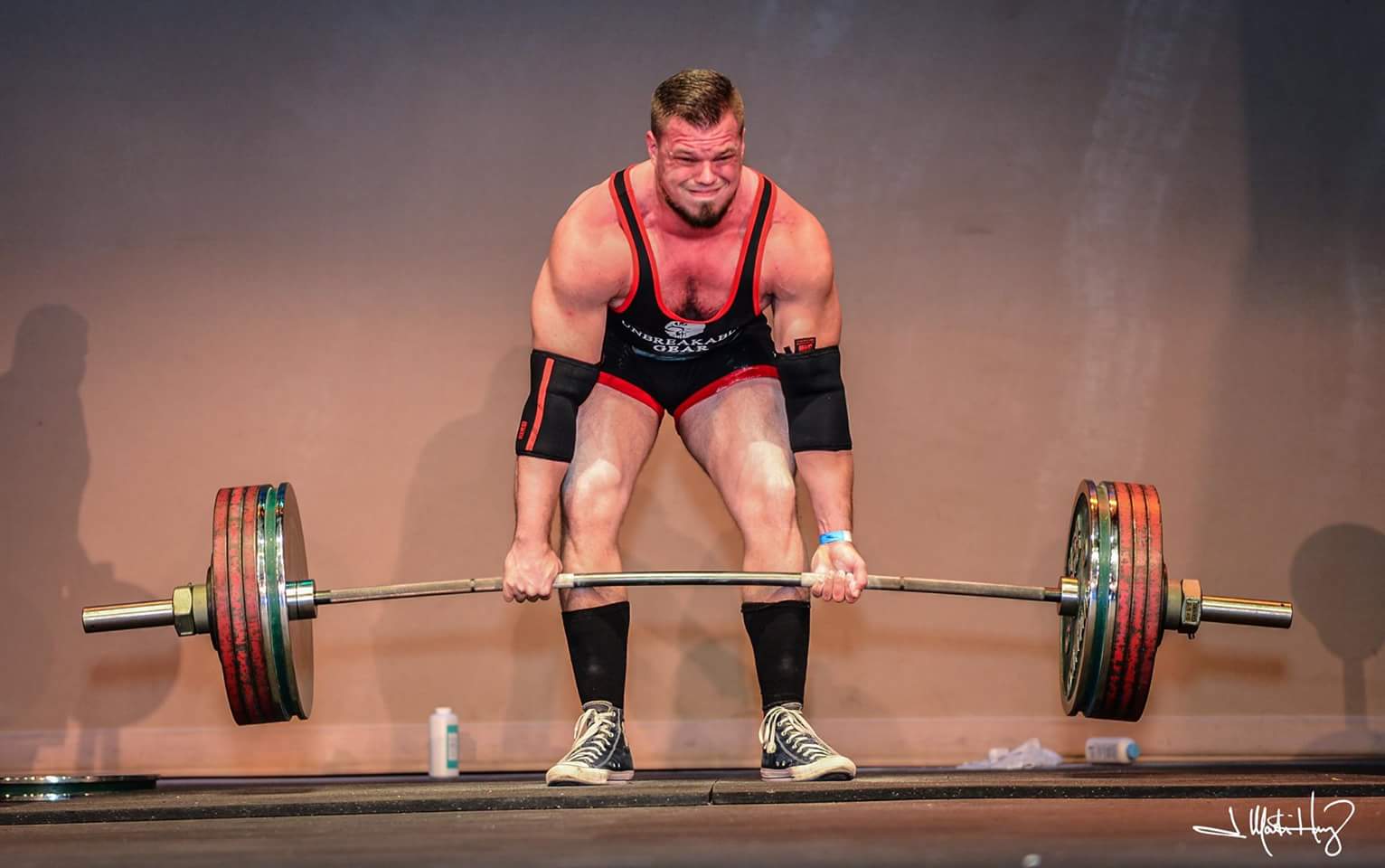
Competitions
- Powerlifting Results (by overall wilks)
- 2016 Southwest Shootout (11th)
- 2016 WABDL push-pull (3rd)
- 2016 Arizona State Powerlifting Championship (3rd)
- 2017 Western Warrior Powerlifting Challenge (6th)
Some Favorite/Interesting
- Papers
- Model Free Techniques for Reduction of High-Dimensional...
- Identification of distributed parameter systems: A neural...
- Reconstruction of normal forms by learning...
- Approximation theory of the MLP model in neural networks
- On Local Homeomorphisms of Euclidean Space
- Books
- Hale & Kocak, "Dynamics and Bifurcation"
- Brunton & Kutz, "Data-Driven Science & Engineering"
- Smoller, "Shockwaves and Reaction-Diffusion Equations"
- Lee, "Introduction to Smooth Manifolds"
- Law, "Data Assimilation: A Mathematical Introduction"
School
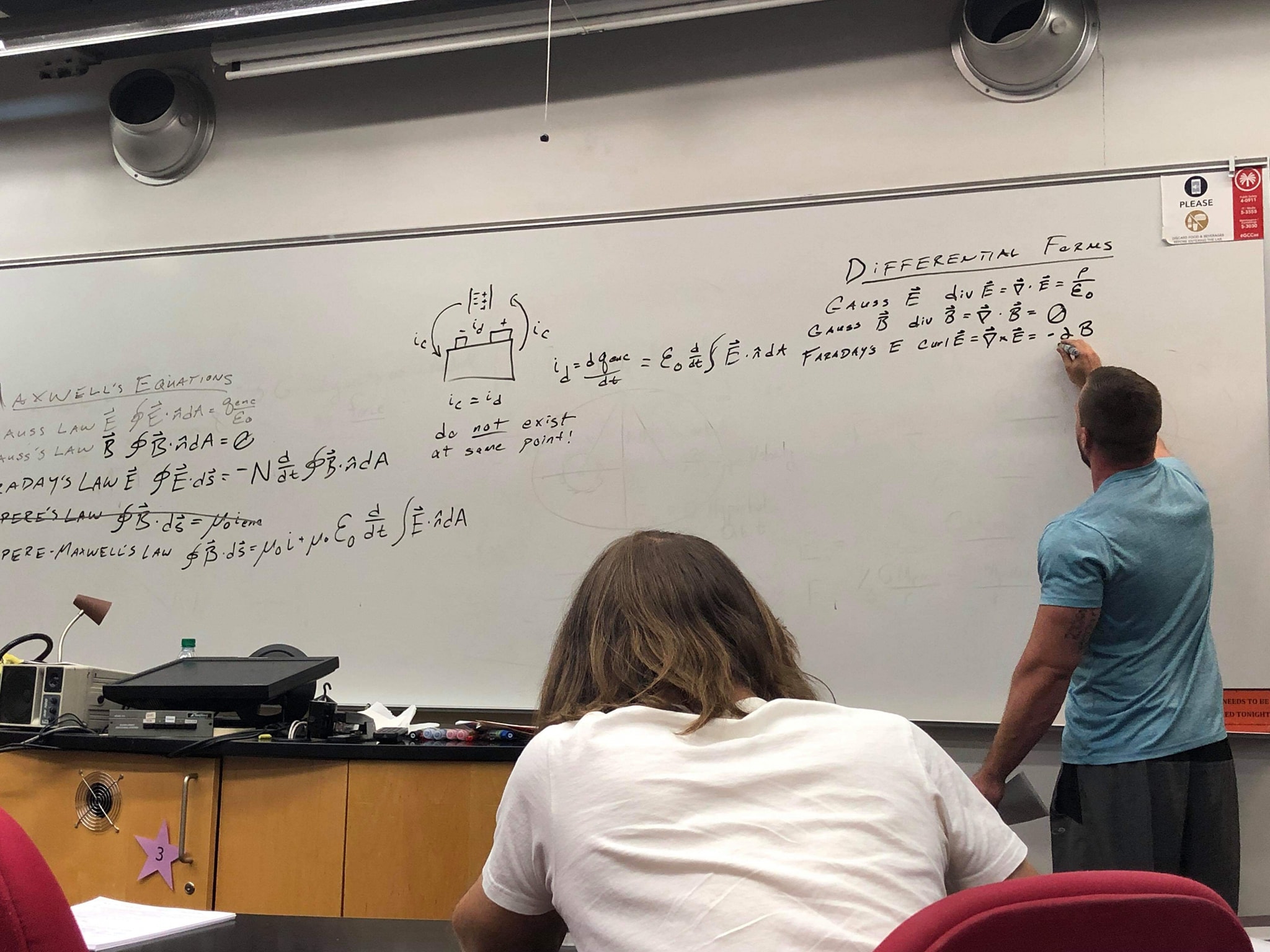
Research Interests
- Dynamical Systems:Model/manifold reductions, Data assimilation, uncertainty and prediction
- Geometry of Data: Diffusion maps, Manifold learning, Kernel learning
- Sparse Recovery: model/manifold recovery, identifiability
Teaching/TA
- Fall 2023
- Math 677: Mathematical Foundations of Data Science
- Spring 2024
- Math 601: Mathematics for Engineers
- Summer 2024
- Math 409: Analysis on the Real Line
Studying/Quals
- Differential Geometry and Manifolds
- Problems in manifolds and Riemannian geometry
- High-Dimensional Probability
- Problems in high-dimensional probability
connect
questions, comments, or concerns? please don't hesitate to reach out.